Artificial intelligence (AI) has long been a present-day topic and is having an impact on the economy, society, skilled work and the work environment. However, there are often very different assessments of the effects: On the one hand the loss of jobs and even professions has been predicted, on the other hand new support and shaping options for work are emerging. In addition, AI is treated as a powerful buzzword without considering the real technologies and requirements behind it. Nevertheless, consequences for the world of work and its employees can only be derived and vocational training concepts designed if the handling of AI in skilled work has been concretized beforehand. The impact of AI on vocational education and training and on the skilled worker has so far been discussed in a rather abstract way and only very rarely focused on research. At the same time, technological developments in certain areas (including expert systems[1], machine learning approaches, digital twins[2]) have already proceeded to such an extent that the effects on skilled work are noticeable and are thus evident. Much will depend on the design of the human-machine interface.
In order to evaluate how skilled labour and AI can successfully “cooperate” in manufacturing, a model is presented here that can support the evaluation process.
Keywords: Vocational Education, Industry 4.0, Autonomy, Automation, Human
1 Preliminary remarks
The article presents a model that provides guidance for describing, deciding, and evaluating AI-supported skilled work. With the help of the model, indications are given as to which contents are relevant for the shaping of skilled labor occupations. The obvious question of new occupations at the skilled worker level is not pursued in the article. To be able to do this, further research is needed. The empirical work available to date tends to see a need for modernization and redesign of existing occupations.
The model is based on the authors’ research in the manufacturing sector. Occupational science methods were used to investigate the requirements for skilled labour and potential applications of AI. The objective of the occupational science research was to survey the requirements for skilled work on the shopfloor. The investigations focused on organizational, technological and social developments related to work and the resulting consequences for skilled work (Spöttl & Windelband 2021).
The findings from the surveys were expanded to include defined autonomy levels for AI (BMWi 2019, 14). Particular attention was paid to
- levels of sophistication in applications of AI and
- possibilities of interaction (technological, organizational, social) with AI at the skilled labour level.
The model is understood as an application model for the interaction of skilled work and AI. Its performance lies in the fact that it can be used to characterize, assess and evaluate the possibilities of AI supported skilled work in manufacturing.
2 Industry 4.0 and the autonomy of technologies
A central question for vocational education and training is: Can technology replace human performance and human intelligence and how does this change qualification structures and learning requirements? There is no doubt that technological development has advanced to the point where large parts of individual areas of skilled work can be influenced or even replaced by automation: Welding robots produce basic body shells almost without human involvement, transport systems in production operate without drivers, and products are linked to local databases or even global (internet) information networks and thus control production processes independently. Thus, while the relief of humans or even the devaluation or replacement of human action by machine action has long permeated our private, social, and professional lives (Brynjolfsson & McAfee 2014), it is becoming increasingly clear that the thus changed and newly emerging worlds of life and work are themselves becoming the subject of skilled work. The automation of manual tasks (especially of repetitive tasks) by robots and increasingly also of more cognitive tasks is leading to sometimes far-reaching changes in employment structures, the required skill profiles, and possibly even occupational structures. So far empirical studies of sociological research are available (Rammert 2016) up to socionics, an interdisciplinary field of research between sociology and AI. The question is explored whether and how it is possible to develop computer programs capable of communication and cooperation based on the model of human society) and labor market research (Dengler & Matthes 2018). However, concrete changes in tasks and competence requirements are still largely unknown. So how are automation, digitization or even artificial intelligence related and how do they change the requirements of skilled vocational work and the vocational training geared to it?
2.1 Industry 4.0 and connectivity
The core of “Industry 4.0” (I40) is the linking of physical components – for example, a machine tool – with components computerized or digitized via the Internet to realize the vision of self-regulating production. Systems linked with software in this way are referred to as cyber-physical systems (CPS) or, with direct reference to production, as cyber-physical production systems (CPPS). CPPS systems dissolve the hierarchical structures of the automation pyramid (VDI & VDE 2013) and integrate people and machines in a production method oriented toward flexibilized production structures with the help of standardization approaches (Acatech 2013).
The increasing networking of “things” is currently creating an “Internet of Things” (IoT). The IoT is used as a term for the infrastructure in which different objects communicate with each other and data are processed directly via data processing (Spöttl & Windelband 2021). Interaction occurs in the sense of data acquisition, data processing and data transport between technical systems. A decisive characteristic of the interaction is the “intelligence” it contains, which is dimensioned by how autonomously the interaction takes place and how appropriate it is for our human coexistence and the real requirements for technical work. Since the autonomous triggering of an interaction presupposes decisions, it is precisely these that are to be scrutinized. While old familiar programs basically incorporate figures and facts with their algorithms, AI goes beyond this and evaluates them.
2.2 Intelligence of technical systems as an object of work
The autonomy of systems with respect to effects that cross system boundaries is a decisive feature for characterizing the intelligence of technical systems and thus of AI. In general, it is very difficult to precisely define the concept of intelligence (Russell & Norvig 2021). Turing’s (1950) characterization still provides the most solid approach to identify “smart systems” (i.e. “intelligent systems” that are able to continuously analyze processes and situations and make predictions and decisions on their own based on the data situation and react accordingly to changing situations). The so-called Turing test consists of checking whether a technical system behaves in such a way that intelligence would be attributed to a human being if it behaved in the same way. Seven characteristics (Becker 2004, 169 f.; Bechtel & Graham 2017; Bechtel, Abrahamsen, & Graham 1998, 10 ff.) are specific for this test:
- Ability to take in, process, and translate information into behavior appropriate for the situation (informing).
- Ability to store functions in a “memory”; retrieval (networking).
- Ability to learn from changing systems and environmental conditions (learning).
- Ability to make appropriate decisions based on changing systems and environmental conditions (decision making).
- Ability to independently plan actions based on experience and characteristics of systems and environmental conditions (planning).
- Increasing communication capability between systems and the human-system complex (communicating) and
- Ability to focus on the environment when acting in systems (interacting).
Associated with these seven characteristics there are active interventions in living and working environments. These are generally associated with intelligence to characterize the effects of “intelligent” action. This also includes intelligent interaction with the environment and social & emotional intelligence (Mahdawi 2017).
When applied to technical systems, these seven characteristics are rarely met without restrictions. Therefore, they should be seen as a continuum of individual and yet at the same time interrelated elements that are evaluated in a comparison between technical and human behavior. Moreover, they provide starting points for the study of skilled work in technology fields permeated by AI.
In technology, the following fields can be distinguished in which AI approaches are applied (Becker et al. 2021):
- Skill-based systems: robotics, transportation systems, Computer Integrated Manufacturing warehouse systems in the field of logistics.
- Knowledge-based systems: expert systems, assistance systems, and agents.
- Learning-oriented systems: Fuzzy logic (a theory developed primarily for representing human knowledge and reasoning for processing in computers), neural networks, machine learning, and model-based methods.
- Simulation-oriented systems: Digital Twins (Ostaševičius 2022).
In further development of the above features, the technology fields mentioned are significant and are taken into account. The seven characteristics can be applied to the individual fields of technology. Whether the people involved in them are ultimately considered to behave intelligently would be a question that still needs to be clarified empirically. However, that is not the intention of this article.
1 A model for acting within the framework of specialized work with artificial intelligence
The further development of automation approaches towards technical realizations of artificial intelligence (AI) is increasingly approaching an implementation in work environments. One example of this is the development of numerous assistance systems. With the help of a model that characterizes “intelligent” interaction in skilled work as acting on and with AI, the authors aim to provide a basis for investigating, describing, and evaluating this acting. The model is also intended to help reintegrate skilled workers (Schaupp 2022, 11 ff.). This should make the intelligent interaction in the specialized work more transparent so that it can be supported in a more targeted manner. It should be noted that artificial intelligence covers the entire continuum from simple information processing to thinking processes (Minsky 1988) on the one hand, and on the other hand also all transfer problems between cognitively shaped and skill-based actions, and highly different professional tasks which are associated with it (Dreyfus et al. 1986).
The so-called learning systems anchored in AI independently find solutions for their defined tasks, among other things by observing their environment and the deriving of rules. A distinction is made between strong and weak AI. “Strong AI” is understood here as a programmed computer that thinks and acts like a human and can ultimately even have consciousness[1]. “Weak AI” is geared toward solving specific tasks in a previously defined area – and only in that area (VDI Technology Center 2018). Industrial-technical professions such as industrial mechanics, machining mechanics, mechatronics engineers, and even production technologists are already confronted with the effects of such intelligence in their everyday work (Spöttl et al. 2016; Becker & Spöttl 2019). The handling of the systems is related to the concrete work tasks.
3.1 Design of a model
The already existing schemes for assessing the permeation or digitalization readiness (i.e., the degree of digital maturity based on various dimensions) of companies and skilled labor tend to be technology driven. Such a restriction to the technology dimension is not expedient. Social, personal, and ethical components as well as values and questions of sustainability must be integrated in the same way and, finally, the actions themselves must be subjected to an evaluation. Even an existing classification of autonomy (BMWi 2019, 13) can only be a first step towards assessing professional action. The classification of autonomy according to publications of the BMWi (2019) already focuses on people (see Table 1). This is an important step for the design of a model.
Table1: Overarching definition of autonomy levels in industrial production not/little influenced by AI (BMWi 2019, 14).
Level 0 | No autonomy, human has full control without assistance. |
Level 1 | Assistance with selected functions, human is always responsible and makes all decisions |
Level 2 | Temporary autonomy in clearly defined areas, human is always responsible and sets (partial) goals |
Level 3 | Delimited autonomy in larger subareas, system warns in case of problems, human confirms proposals for solution of the system or acts as fallback level |
Level 4 | The system works autonomously and adaptively within certain system limits, human can monitor or act in emergency situations |
Level 5 | Autonomous operation in all areas, including cooperation and changing system boundaries, human can be absent. |
In the end, the greatest challenge lies in mastering the complexity of these systems. Today, specialists try to make their decisions based on experience in maintenance, among other things, and use intuition, feeling, sentiment and the evaluation of various process data for this purpose (Dreyfus & Dreyfus 1986; Böhle 2017). In the case of AI-based systems, the question arises as to which intervention options remain for skilled workers and which (new) tasks arise when using such systems (Schaupp 2022). This is known by the term “automation dilemma” (Bainbridge 1983): In increasingly automated systems, the building up and use of expert knowledge on the part of humans is made more difficult or is even prevented. At the same time, however, there is a need for skilled workers to maintain the ability to act and make decisions to identify errors and to develop possible solutions in crucial situations. At the same time, depending on the training occupation, skilled workers must learn how to analyze relevant data and to evaluate and process them in relation to the situation. In this respect, a model is required that can be used to describe the actions of specialists in and on AI-influenced systems. It should be noted that there is no such thing as “AI”: In reality it always encompasses a continuum of the five analytically described levels (see Table 1).
An extension of the labeling of an Industry 4.0 penetration in the two technologically dominated perspectives of “product” and “production” (as focused by the VDMA[1] classification) is achieved by labeling work requirements. The latter are brought into an action context in production or in dealing with the product. In this way, action spaces and changed work processes become clear from the point of view of the skilled workers. This results in a classification of the permeation of action spaces of skilled workers by CPS and AI. The five levels of autonomy (see Table 1) are linked to the interaction of skilled workers with the respective degree of AI expression and the concrete product and process technologies of Industry 4.0. The decisive factor here is the interaction of skilled workers with the respective degree of expression, which in turn is characterized by the emerging human-machine collaboration. After all, it is the mechanism of artificial intelligence that once automate skilled worker actions or change processes so that skilled workers have to deal precisely with these changed processes. Automation with the help of artificial intelligence (Hirsch-Kreinsen & Karačić 2019) thus becomes the object of action of skilled work, either the realization of automation itself or the precise work process changed by automation (Schaupp 2022, 7 ff.).
3.2 Levels of a model
Level 1 is characterized by the fact that information about a product or a process can be attached to an object and specialists are confronted with exactly that. In automated assembly systems, for example, they are challenged to view order data, production data and delivery data along with the product and, if necessary, to relate them to planned production processes. Specialists are confronted here with a new variant of information transport and must be able to deal with it, both in the sense of processing the information and by using an intelligent procedure in the transport of data and information.
If the object or asset itself has a form of artificial intelligence, i.e., if it is equipped with a microprocessor and a program with corresponding logic, this results in Level 2 ofthe manifestation of CPS. Such a constellation is called an embedded system (an embedded system is a digital system – also called a computer system – that is embedded in and interacts with a surrounding technical system). Stage 1 capabilities are embedded in the object itself and no longer tacked on. Industrial products based on embedded systems can be supplied with different data and thus acquire different properties. In addition, depending on the interfaces available on the embedded systems, there are possibilities for the automated transfer of information to other parts of the plant. Specialists then carry out tasks of coding (adaptation in relation to a certain configuration), parameterization (provision of the object with a certain property) or monitoring of self-regulation (automated passing on of information to another plant part).
Level 3 of the CPS characteristic consists of the interaction between tools, computers, and systems. In other words, “things” with Level 2 properties independently pass on information in both directions depending on system properties or triggered by predefined time intervals or “jobs” and automatically change the way the production system functions. Networking between the mold and the system enables, for example, system monitoring, fault diagnosis or remote maintenance. One example is the CAD-CAM (Computer Added Design – Computer Added Manufacturing) coupling in workshop production with connection to quality assurance systems, for example to record and document wear data of tools.
In Level 4, production data are used to influence production processes. A smart meter (a smart meter is an intelligent measuring system) with a digital and internet enabled measuring system and a communication unit (gateway). This allows to communicate current consumption data to be queried by the user, and feeds electrical energy into the grid depending on the electricity price. In case of reports of a reduction of the wear and tear stock of a machine, the respective tool is ordered automatically. Such interactions must be monitored, installed and configured by skilled personnel and, if necessary, adapted to changed production environments.
Finally, Level 5 corresponds to the actual vision of Industry 4.0. With the inclusion of human beings, things (tools, plants, systems) are assigned “intelligent” properties so that the overall system or process regulates itself as far as this is technically feasible. The extent to which this stage will generate new tasks for skilled workers and which role skilled workers are still likely to play is currently least foreseeable and represents a company-specific and even a societal design task.
Table 2: Model for describing levels of skilled work on and in AI-influenced (production) systems (based on Becker 2016, 74; Dreyfus & Dreyfus 1986).
Expression level of artificial intelligence | Central technology feature / example | Role of the humans/ of skilled workers | Work requirements: interaction with AI as the subject of skilled work | |
1 | Information storage Information processing external (assistance: data autonomy). | Product with data storage / RFID chip (RFID stands for Radio Frequency Identification) | Operation with externally available data | Handling of order data directly related to the product, production data, delivery data, service data |
2 | Embedded system (system immanent autonomy: information processing internal) | Information processing in the product and process / microprocessor in the subsystem | Operation with internally available data | Realization of coding, parameterization and self-regulation of plant components |
3 | Communication (delimited autonomy) | Internet interface / field buses; OPC (OPC stands for Open Platform Communications) | Communication across interfaces and disciplines (in case of a need for clarification) | Addressing the transfer of information between sensors, actuators, tools, computers, and equipment. |
4 | Interaction (cross-system autonomy) | Internet-based communication and triggering of “actions” / smart grids (intelligent power grids) | Monitoring of complex processes, intervention in case of malfunction and communication with various functional units | Realization of and dealing with product and process influence by machines, plants and production systems |
5 | Cooperation & Collaboration (self-sufficient autonomy) | Vision of self-regulating production / digital factory | Role of humans still open – not yet clarified whether only simple monitoring or (co)design of the processes | Designing forms of cooperation between man and machine |
The need to significantly expand the technology-oriented view of products and processes by taking into account the autonomy of the resulting human-machine systems as such and in particular as a work object of skilled professional work becomes apparent. This step is a basic condition for human-centered production and assembly. This is what makes socio-technical work design possible in the first place.
4 Example of professional technical work on and with artificial intelligence
Numerous examples of working with and on AI-supported systems could now be cited (Becker, Spöttl, & Windelband 2021, 45 ff.), one of which should serve as an example to demonstrate the “new” character of specialized work quite clearly: Working with digital twins.
Digital twins (Ostaševičius 2022) are already in use in production and are a further development of simulation programs. They can reflect holistic images of reality including all physical properties: in the area of predictive maintenance, in energy data management, in resource optimization or production planning for trouble-free production. Specialists are working at the digital copy of a plant just as they would on the physical plant to put it into operation, determine maintenance requirements, and reduce energy and material consumption. One application example is the widely used automation environment developed by Siemens with NX (NX is a fully three-dimensional system with double precision that enables the exact description of almost any geometric shape) and the TIA Portal (Totally Integrated Automation Portal: it enables complete access to the entire digitized automation). This which brings together various simulation and control programs for production (Siemens 2018). In the future, the handling of “Internet operating environments” such as MindSphere (MindSphere is an industrial IoT service solution that uses sophisticated analytics and AI to implement IoT solutions from Edge to Cloud) will also be part of these tasks. However, in the case of digital twins, the abstraction and the theory-oriented approach sometimes reach a level which, at least at present, still primarily requires academically trained specialists.
The switch between work in virtuality and reality is becoming increasingly commonplace in skilled work due to digital twins. It is remarkable that it is the human being who must design and monitor this switch and interactivity. Undoubtedly, this switch can also be automated and thus devalue human work. It remains a design task to shape it in a way that an overall social acceptance prevails (Noble 2011). Examples observed by the authors in their technical work indicate that, similarly to the automation dilemma, it is difficult, if not impossible, to ignore the human capacity for action. A very simple example: A fully automated production line for the manufacturing of ball bearings is not able to consider changed conditions, for example, outer rings which have started to rust during their intermediate storage. Despite full automation, it is the human being who determines measurements for a “fully automated” production based on experience.
1 Conclusion
The model can be helpful for the description, assessment and evaluation of AI-supported skilled work. The advantage of such a model is that skilled work can be classified and evaluated even when used in highly complex systems because professional competencies can be described via the different levels of expression of artificial intelligence. In this feature, it can be seen as a reference for planning qualification initiatives. Above all, false conclusions for the design of industrial-technical training regulations and framework curricula are to be avoided by examining the vocational action sequences with the help of the model more closely in terms of content. In doing so, it is not only important to consider technological changes, but also the shifts in communication, cooperation and collaboration structures due to the organization of process flows. Influenced by AI, changed competence requirements arise for professionally qualified persons who process tasks in partially completely changed work organization structures.
For vocational training, some open questions can be derived that will be relevant in the future:
- Does the influence of AI create independent new, or rather integrative and changed competence requirements?
- The increasing autonomy of technology, also with an effect on action structures, leads to the replacement of occupational competences, certain occupational qualification and possibly even entire professions. As with the question of the automation dilemma (Bainbridge 1983), the question of the autonomy dilemma[1] is now arising under the influence of AI: If competences, experiences and decision-making bases for certain contexts of action are “absorbed” into machines, how can these then be prepared for learning processes and transferred to subsequent generations of skilled workers’?
- Will the influence of AI-supported technologies lead to the emergence of new professions or new types of professions, such as hybrid professions? Following the introduction of production technologists, which arose rather from the idea of an optimized production organization, considerations are now in the center of interest aiming at replacing industrial mechanics with asset managers (in the Industry 4.0 context, an asset is any virtually mapped object that has a value for an organization. Simply put: any object in the physical world that has a connection to the internet). The basis for such a job profile would be an empirically identifiable range of tasks that would stand up to the criteria for a profession (VDI & VDE 2013).
- What needs to be investigated are the concrete changes in skilled work due to the changed role of technology and the resulting qualification requirements. Only in this way useful descriptions can be created for the design of occupational profiles and training regulations.
- What do the new and changed professional tasks and competence requirements due to AI- influence look like in the four fields (see Figure 1), which are raised by the two dimensions “information processing” and “autonomy”? And can the hypotheses assigned to the fields be confirmed? (Rohrbach-Schmidt & Tiemann 2013)
The model for the characterization of skilled work in connection with AI, which was developed on the basis of various approaches to the design of industrial and manual work, requires supplementary empirical validation for further verification. The following hypotheses (see Fig. 1) can form the basis for it.
Hypothesis 1: A low degree of information processing and a low autonomy of technology lead to a relief of occupational tasks by machines.
Hypothesis 2: A high degree of information processing and a low degree of autonomy of technology lead to an increase in occupational tasks.
Hypothesis 3: A low degree of information processing and a high degree of autonomy of technology lead to a substitution of occupational tasks by machines.
Hypothesis 4: A high degree of information processing and a high degree of autonomy of technology lead to a high demand for occupational tasks unless they are not algorithmic in nature and to a low demand for occupational tasks if they are algorithmic in nature.
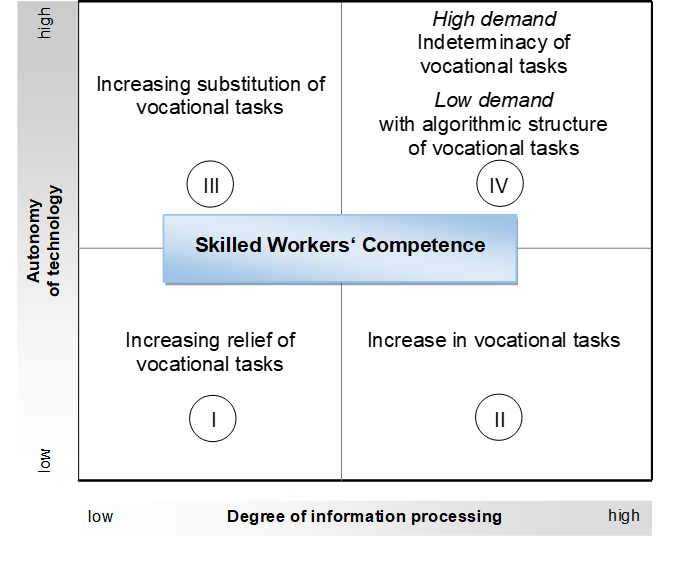
The four hypotheses provide a basis for discussion. The aim is to clarify
- how far the autonomy of technology is likely to develop and
- what level of information processing is likely to be achieved.
Depending on the results, considerations can be made about the design of the qualification of skilled workers.
A further step can be the optimization of the model in order to have a tool available for dealing with AI that can be used to assess, describe and evaluate AI-supported skilled work.
[1] An expert system provides people with solutions for complex problems in a limited specialist area. It acts as an expert, so to speak, and provides support in the form of recommendations for action.
[2] A digital twin refers to a computer-based model of a tangible or intangible object that can replicate as many of the properties of the physical object as possible (Ostaševičius 2022).
[3] In the field of artificial intelligence, the concept of consciousness expresses the fact that the perceived environment and the input data can be combined in the computer programs to form a representation of reality that conveys “meaning”. This is calculated by the AI and must be measured against what humans would define as meaning in such a context (Schaupp 2022).
[4] VDMA (Verband Deutscher Maschinen- und Anlagenbau e. V.): With over 3400 members, the VDMA is the largest network organization and an important voice for the mechanical engineering industry in Germany and Europe.
[5] Following the debate about an increasingly supervisory role of skilled workers due to an increase in automation, Ohno (1988) coined the term “autonomation” for the opposite school of thought about the increasing autonomy of machines.
References
Bainbridge, L. (1983). Ironies of Automation. In: Automatica, 19, 6, 775-779.
Bechtel, W. & Graham, G. (2017). A Companion to Cognitive Science. Oxford: Wiley.
Bechtel, W., Abrahamsen, A., & Graham, G. (1998). The Life of cognitive science. In: Bechtel, W. & Graham, G. (eds.): A Companion to Cognitive Science. Oxford: Wiley, 1-105.
Becker, M. & Spöttl, G. (2019). Effects of digitalization on vocational education using the example of the metal and electrical industry. In: Journal of educational science, 21. Wiesbaden: Springer, 567-592.
Becker, M. (2004). KI – Optimierung der Diagnosearbeit oder Beitrag zur Dequalifizierung von Kfz-Mechatronikern? In: Becker, M., Schwenger, U., Spöttl, G., & Vollmer, T. (eds.): Metallberufe zwischen Tradition und Zukunft. Bremen: Donat, 167-181.
Becker, M. (2016). Work processes and vocational training in the context of “Handwerk 4.0”. In: Jaschke, S., Schwenger, U., & Vollmer, T. (eds.): Digitale Vernetzung der Facharbeit. Gewerblich-technische Berufsbildung in einer Arbeitswelt des Internets der Dinge. Bielefeld: W. Bertelsmann, 43, 71-86.
Becker, M., Spöttl, G., & Windelband, L. (2021). Künstliche Intelligenz und Autonomie der Technologien in der gewerblich-technischen Berufsbildung. In: Seufert, S., Guggemos, J., Ifenthaler, D., Ertl, H., & Seifried, J. (eds.): Künstliche Intelligenz in der beruflichen Bildung. Zukunft der Arbeit und Bildung mit intelligenten Maschinen?! Stuttgart: Franz Steiner Verlag. Zeitschrift für Berufs- und Wirtschaftspädagogik – supplement 31, 1, 31-54.
BMWi (2019). Technologieszenario “Künstliche Intelligenz in der Industrie 4.0”. Berlin: Federal Ministry for Economic Affairs and Energy. Online: https://www.plattform-i40.de/PI40/Redaktion/DE/Downloads/Publikation/KI-industrie-40.html (retrieved: 26.02.2020).
Böhle, F. (2017). Arbeit als Subjektivierendes Handeln. Agency in the face of imponderables and uncertainty. Wiesbaden: Springer.
Brynjolfsson, E. & McAfee, A. (2014). The second Machine Age. How the next digital revolution will change all our lives. New York: W. W. Norton & Company.
Dengler, K. & Matthes, B. (2018). Substitutability potentials of occupations. Few occupational profiles keep pace with digitalization. IAB Short Report. Online: http://doku.iab.de/kurzber/2018/kb0418.pdf (retrieved: 03.05.2020).
Dreyfus, H. L. & Dreyfus, S. E. (1986). Mind over Machine. The Power of Human Intuition and Expertise in the Era of the Computer. New York: The Free Press.
Hirsch-Kreinsen, H. & Karačić, A. (2019). Autonomous Systems and Work. Perspectives, challenges and limits of artificial intelligence in the world of work. Bielefeld: transcript Verlag.
Mahdawi, A. (2017). What jobs will still be around in 20 years? Read this to prepare your future. In: The Guardian. Online: https://web.archive.org/web/20180114021804/https:/www.theguardian.com/us-news/2017/jun/26/jobs-future-automation-robots-skills-creative-health (retrieved 13.01.2020).
Minsky, M. (1988). The Society of Mind. New York: Touchstone.
Noble, D. F. (2011). Forces of Production: A Social History of Industrial Automation. New Brunswick: Transaction Publishers.
Ohno, T. (1988). Toyota production system: Beyond large-scale production. New York: Productivity Press.
Ostaševičius, V. (2022). Digital Twins in Manufacturing: Virtual and Physical Twins for Advanced Manufacturing. Heidelberg: Springer.
Rammert, W. (2016). Technology – Action – Knowledge. Towards a pragmatist theory of technology and social theory. Wiesbaden: Springer.
Rohrbach-Schmidt, D. & Tiemann, M. (2013). Changes in workplace tasks in Germany: Evaluation skill and task measures. In: Journal of Labour Market Research, 46, 3, 215-237.
Russell, S. & Norvig, P. (2021). Artificial Intelligence: A Modern Approach. Hoboken: Pearson.
Schaupp, S. (2022). Cybernetic proletarianization: Spirals of devaluation and conflict in digitalized production. In: Capital & Class, 46, 1, 11-31.
Siemens (2018). CNC4You. Praxiswissen für die Werkstatt. Online: https://static.dc.siemens.com/cnc4you/magazines/cnc4you_2018_2_de.pdf (retrieved 16.05.2022).
Spöttl, G. & Windelband, L. (2021). The 4th Industrial Revolution – Its Impact on Vocational Skills. In: Journal of Education and Work, 34, 1, 29-52.
Spöttl, G., Gorldt, C., Windelband L., Grantz, T., & Richter, T. (2016). Industrie 4.0 – Auswirkungen auf Aus- und Weiterbildung in der M+E-Industrie. Munich: Bayerischer Unternehmensverband Metall- und Elektro e.V. (bayme) & Association of the Bavarian Metal and Electrical Industry e. V. (vbm). Online: https://www.baymevbm.de/Redaktion/Freizugaengliche-Medien/Abteilungen-GS/Bildung/2016/Downloads/baymevbm_Studie_Industrie-4-0.pdf (retrieved 21.06.2022).
Turing, A. (1950). Computing Machinery and Intelligence. In: Mind, 59, 236, 433-460. Online: http://www.jstor.org/stable/2251299 (retrieved 11.05.2022).
VDI & VDE (2013). Cyber-Physical Systems: Opportunities and Benefits from an Automation Perspective. VDI/VDE Society for Measurement and Automation Technology.
VDI Technology Center (2018). Innovation update. Digitalization: Continuing education and AI. Online: https://www.vditz.de/publikation/digitalisierung-weiterbildung-und-ki-im-fokus/ (retrieved 24.02.2022).